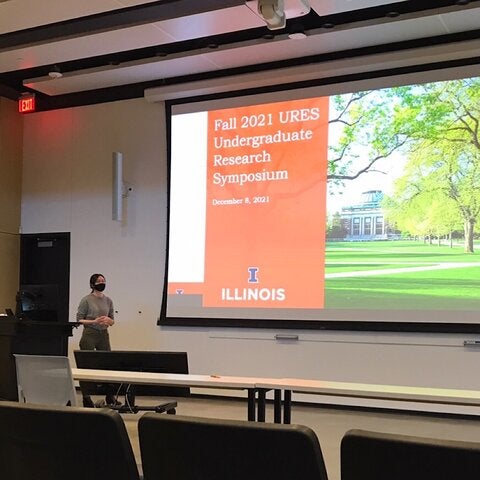
Undergraduate students in the Department of Statistics presented research projects to peers, mentors, and general attendees at the Fall 2021 Undergraduate Research Experience in Statistics (URES) Symposium, held on December 8, 2021. Paired with faculty mentors, students selected for the URES program collaborate and develop projects throughout the semester in preparation of presenting their findings at the symposium. Projects vary and can range from a variety of topics such as baseball data analytics, climate change, genomics, network data analysis, programming methods, public health, spatial statistics, survival analysis, and many more.
Undergraduate students in either the Statistics major or Statistics & Computer Science major are welcome to apply to the URES program at the beginning of each term. Students may be selected to assist on a current project that a faculty mentor has in progress or they may choose a topic for research. The symposium was held in the Campus Instructional Facility, which gave students the opportunity to present their research at the front of the classroom in the new state-of-the-art facility.
A complete list of presenters, mentors and their topics is listed below.
Analysis of psychometric data using Bayesian inference of latent classes - Daniel Huang, Adrian Pizano, Scott Turro, Hanling Zhang mentored by Steve Culpepper
Abstract: We consider latent class models (LCM) for multivariate binary response data, which is common in psychometric research. LCM are useful for identifying groups of individuals who respond in similar ways. We employ Gibbs sampling to estimate the distribution of the parameters, a typical technique for Bayesian algorithms. We provide a python package and R functions implement our algorithm and show the accuracy with a Monte Carlo simulation on test data. We apply our technique to various psychometric datasets and analyze the results.
Parallel computing for variance reduction of estimates of expected Darwinian fitness - Sicong He mentored by Daniel Eck
Abstract: Precise estimation of expected Darwinian fitness, the expected lifetime number of offspring of organism, is a central component of life history analysis. The aster model serves as a defensible statistical model for distributions of Darwinian fitness. The aster model is equipped to incorporate the major life stages an organism travels through which separately may effect Darwinian fitness. Envelope methodology reduces asymptotic variability by establishing a link between unknown parameters of interest and the asymptotic covariance matrices of their estimators. It is known both theoretically and in applications that incorporation of envelope methodology reduces asymptotic variability. Current software which incorporates envelope methodology into the aster estimation framework is too slow and too complicated for users. We develop new software which addresses these shortcomings.
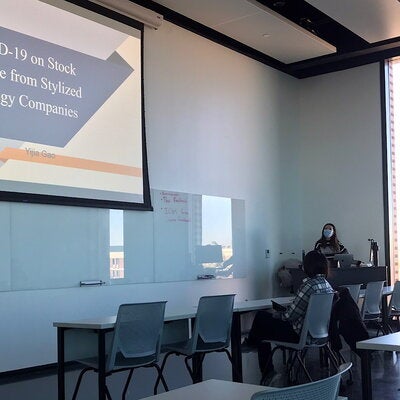
Impact of COVID-19 on the Stock Market - Yijia Gao mentored by Hyoeun Lee
Abstract: In this project, we investigate the impact of COVID-19 on technology company stock prices. We present a set of stylized empirical facts using statistical analysis of normalized returns and volatility of normalized returns based on the stock data of 34 US technology companies. We investigate the impact of COVID-19 on the statistical properties of those companies and we are interested in determining if those stylized facts remain true in light of the events surrounding COVID-19.
Analyze environmental drivers of the biting behavior for several species of malaria parasites mosquitoes in a remote Amerindian region in South America - Songyuan Wang, Carol Song mentored by Lelys Bravo de Guenni
Abstract: Our project goal is to analyze the impact of environmental drivers on the biting behavior for several species of malaria parasites mosquitoes in a remote Amerindian region in South America. This project has been developed in different phases. In phase 1 we analyzed the effectiveness of mosquitoes’ traps baited with two chemical attractants (Octenol and Lurex) based on data collected during year 2015 using a non-parametric ANOVA analysis. In phase 2, we analyzed the impact of different climate predictors on mosquito abundance, including the implementation of different imputation methods for missing data on the number of mosquitoes collected. A next phase of the project attempts to model the impact of climate variables and mosquito abundance on the number of malaria cases using a generalized time series modeling approach.
Application of Bayesian variable selection to college admission data - Abe Sun mentored by Steve Culpepper
Abstract not provided at time of publication
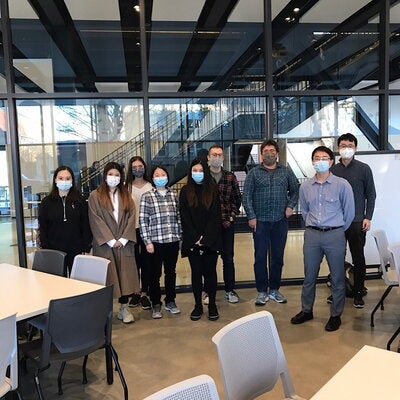
Improvements to SEAM methodology for player matchup evaluations - Julia Wapner mentored by Daniel Eck
Abstract: We further develop the SEAM (synthetic estimated average matchup) method for describing batter versus pitcher matchups in baseball, both numerically and visually. We first estimate the distribution of balls put into play by a batter facing a pitcher, called the spray chart distribution. This distribution is conditional on batter and pitcher characteristics. These characteristics are a better expression of talent than any conventional statistics. Many individual matchups have a sample
size that is too small to be reliable. Synthetic versions of the batter and pitcher under consideration are constructed in order to alleviate these concerns. Weights governing how much influence these synthetic players have on the overall spray chart distribution are constructed to minimize expected mean square error. Current implementation of the SEAM method has shortcoming on several fronts. Through testing and evaluation several enhancements and issues were discovered. Improvements were made to validation of results, computational speed, code dependency, and conceptual implementation.
Sales price prediction using deep learning methods - Baoyu Li mentored by Hyoeun Lee
Abstract: In this research, we focus on time series prediction using deep learning methods. First, we predict the sales price using several deep learning models, such as MLP, RNN, LSTM. In addition, we predict the sales price using a hybrid model, which is a combination of a traditional time series model (ARIMA) and a deep learning model. Finally, we compare the single deep learning method and the hybrid model method, focusing on whether the hybrid model can improve the prediction of the sales price.
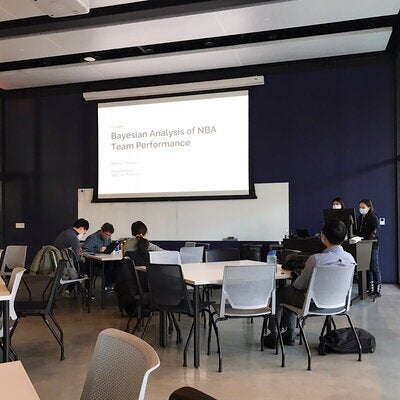
Bayesian Analysis of NBA Team Performance - Jiaqi Hu, Yanyi Lu mentored by Xinran Li
Abstract: According to the SGMA’s U.S. Trends in Team Sports research, there are over 26 million people in the United States who play basketball. And this brings basketball to one of the most prevailing sports among the United States, even the world. Since the establishment of the National Basketball Association (NBA), the public’s enthusiasm for basketball continues to grow, and the popularity of it remains high all the time. At the same time, the public is becoming more concerned about the performance of their favorite team each season. Such circumstances had brought our interest to study the performance of teams in each game season. Previous studies have unveiled some factors that might affect team performance, such as whether teams have home-court advantages, replacement of coaches, time spent on rest, etc. In our study, we will use a dataset containing the data behind the complete history of the NBA from Kaggle, in order to build a model to analyze the performance of NBA teams using statistical methods such as Bayesian Hierarchical Modeling. Our findings suggest that the major factor that contributes to the significant drop/rise of team performances in each season is the replacement of players, such as whether the player is retired or quit the game because of injuries.
Spatial modelling of water quality from multiple pollution sources in the BCE Cambodia wetland using a Bayesian approach - Weain Yin mentored by Lelys Bravo de Guenni
Abstract: This project is a continuation of the project 'Assessing water quality spatial heterogeneity from multiple pollution sources in the BCE Cambodia wetland'. The objective of the project is to characterize the spatial variability of contaminants in the wetland region using Bayesian kriging methods. The water quality data is grouped by rain season and water type (surface water or ground water). Empirical and theoretical semi-variograms are computed for each contaminant to assess the strength of the spatial autocorrelation. It is found that each contaminant exhibits a differentiated spatial dependence structure. Spatial prediction using Bayesian kriging models is being implemented using the R package 'spBayes'.
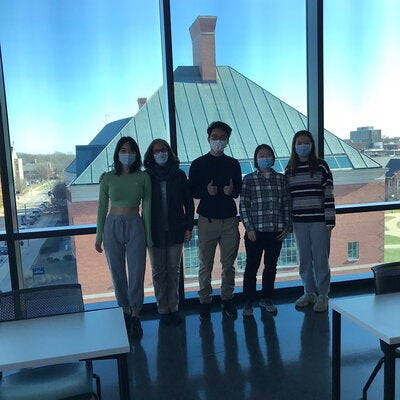
Quantifying climate change uncertainty in Champaign County - Linjia Feng, Tymon Duchnicki mentored by Lelys Bravo de Guenni
Abstract: The NASA Earth Exchange Downscaled Climate Projections (NEX-DCP30) data is used to evaluate future projections of precipitation for the period 2069-2099 from 31 different General Circulation Models (GCMs), under two different Representative Concentration Pathways (RCPs) depicting future greenhouse gases concentration trajectories. The aim of the analysis is to quantify the contribution of GCMs model uncertainty and RCPs into the estimation of the relative change of future precipitation relative to a historical reference period 1950-2005 using linear mixed effect models. Different climate projections produced by the 31 GCMs account for the internal climate variability under the two RCPs scenarios.
Analysis of Midwest Drought Conditions vs. El Niño - Yinmeng Lai mentored by Lelys Bravo de Guenni
Abstract: With farming being a crucial industry in Illinois, understanding drought behavior could help increase foresight in this critical sector of Illinois’ economy. Inspired by previous works done around El Niño and drought episodes, we wanted to analyze the relationship between Urbana’s drought data and El Niño events. Using El Niño indicators Niño 3.4 and Oceanic Niño Index (ONI) data found on noaa.gov, and drought indicators Palmer Drought Severity Index (PDSI) and Self-calibrated Palmer Drought Severity Index (SC-PDSI), we were able to analyze the relationship between drought and El Niño in both, the frequency domain and the time domain. By performing coherence analysis, we were able to find a weak coherence between El Niño data (ONI) and drought data (PDSI). We also produced a one-month ahead prediction model of drought data (PDSI) from El Niño data (ONI) using transfer function models.
Comparison of differential abundance test in compositional data - Jennings Cheng mentored by Shulei Wang
Abstract: Compositional data naturally arise in a wide range of biomedical applications such as microbiome study. Differential abundance test is an essential tool in these biomedical applications. In this project, we compare several different popular differential abundance tests on simulated data set.
Power optimization for knockoff filters - Lauren Bome Jang, Elina Mehra mentored by Jingbo Liu
Abstract: The knockoff filter is a variable selection method developed by Barber and Cand`es 2016 with the purpose of controlling the false discovery rate at an exact threshold. It has since been the basis to several other knockoff methods. We specifically looked at effective signal deficiency (ESD) introduced by Liu and Rigollet 2019. ESD shows that the conditional independence structure of the predictors plays an important role in consistency and hence predicts the consistency of the different variable selection methods. We attempted to optimize the function in the correlation matrix of the predictive variables to control the power of the method.
For more information on the URES program and how you can get involved, click here.