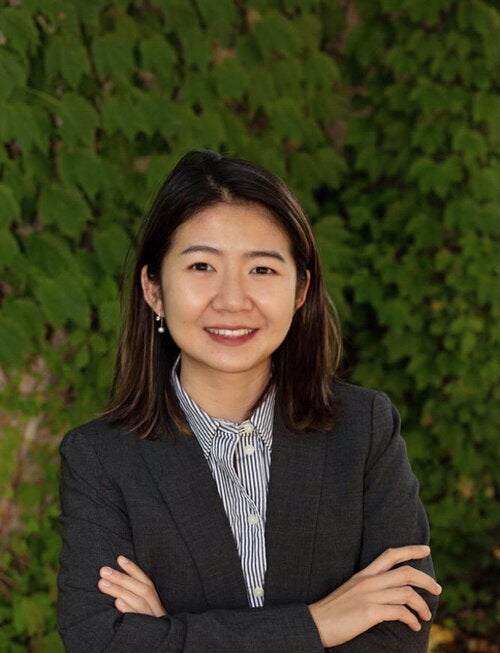
Research Interests
My research focuses on Bayesian theory and methodology, and their intersection with deep learning. In particular, my recent work examines simulation-based inference with generative AI models.
Education
Econometrics and Statistics, PhD, University of Chicago Booth School of Business
Additional Campus Affiliations
Assistant Professor, Statistics
External Links
Recent Publications
Wang, Y., Polson, N., & Sokolov, V. O. (2023). Data Augmentation for Bayesian Deep Learning. Bayesian Analysis, 18(4), 1041-1069. https://doi.org/10.1214/22-BA1331
Wang, Y., Kaji, T., & Rockova, V. (2022). Approximate Bayesian Computation via Classification. Journal of Machine Learning Research, 23, Article 350.
Liu, Y., Ročková, V., & Wang, Y. (2021). Variable selection with ABC Bayesian forests. Journal of the Royal Statistical Society. Series B: Statistical Methodology, 83(3), 453-481. https://doi.org/10.1111/rssb.12423
Wang, Y., & Ročková, V. (2020). Uncertainty Quantification for Sparse Deep Learning. Proceedings of Machine Learning Research, 108, 298-308.