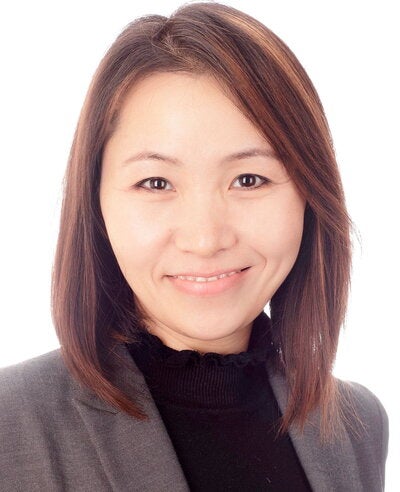
Bohrer Lecture: Judy Wang, George Washington University
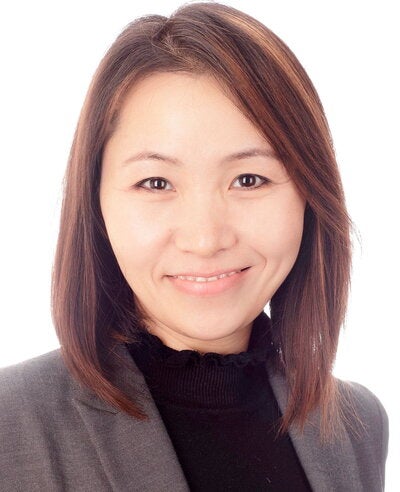
BIO: Huixia Judy Wang is a statistician who works as a professor of statistics at George Washington University. Topics in her research include quantile regression and the application of biostatistics to cancer. Wang graduated from Fudan University in 1999 and earned a master's degree from Fudan in 2002. She completed her Ph.D. in statistics in 2006 from the University of Illinois at Urbana–Champaign. Her dissertation, Inference on Quantile Regression for Mixed Models with Applications to GeneChip Data, was supervised by Xuming He. She joined the statistics faculty at North Carolina State University in 2006 and moved to George Washington University in 2014. From 2018, she has been serving as program director for the Statistics Program at the National Science Foundation.
Talk Title: Flexible Probabilistic Prediction for Non-Gaussian Spatial Processes
Talk Abstract: Many existing methods for analyzing spatial data rely on the Gaussian assumption, which is violated in many applications such as wind speed, precipitation and COVID mortality data. In this talk, I will discuss several semiparametric approaches for probabilistic prediction of non-Gaussian spatial data. First, I will present a copula-based multiple indicator kriging (CMIK) model for the analysis of non-Gaussian spatial data. The algorithm is computationally simple since it models the marginal distribution and the spatial dependence separately. Next, I will introduce a deep multiple indicator kriging (DMIK) method for univariate and bivariate spatial processes. The method is based on thresholding the spatial observations at a given set of quantile values within a deep neural network framework. The DMIK method overcomes the computational challenge in the CMIK for analyzing large datasets. The developed methods do not require any parametric assumptions on the marginal distribution and are thus more flexible than Gaussian-based methods. These methods will also provide convenient ways to construct both point and interval predictions based on the estimated conditional quantiles. Finally, I will briefly discuss semiparametric approaches for analyzing spatio-temporal and count spatial data, and present some numerical results.