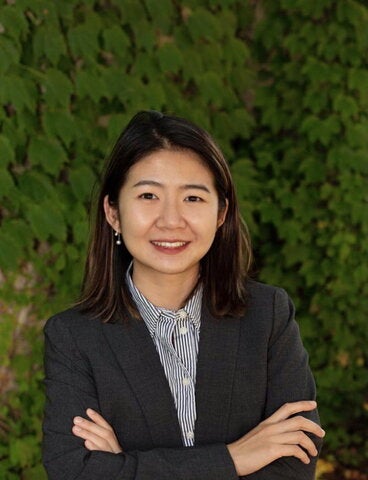
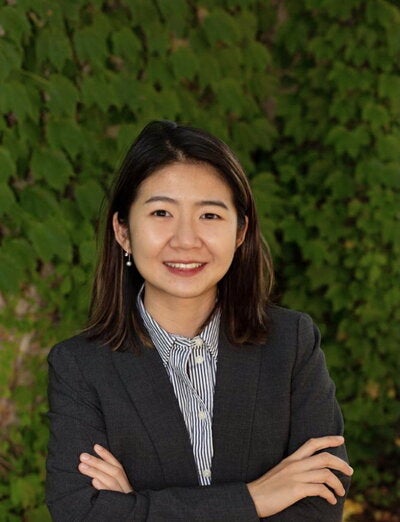
Yuexi Wang, Assistant Professor for the Department of Statistics at the University of Illinois Urbana-Champaign, is revolutionizing how we analyze complex data by merging Bayesian statistics with cutting-edge deep learning. She builds scalable inference tools for real-world challenges, from healthcare diagnostics to economic policy, while training the next generation of data scientists to think beyond predictions.
How did you initially get drawn to the field of statistics?
I did my undergrad in mathematics back in China. There's this cultural thing where being good at math is seen as a strong indicator of intelligence. But during my studies, I realized I wasn't really interested in pure theoretical mathematics — I wanted to work with statistical models that could solve actual applied problems. That's what led me to pursue a master's in statistics at the University of Chicago. During my master's, I met my PhD advisors who really got me interested in research. I ended up staying at UChicago for my PhD but moved from the Statistics Department to the Business School, which gave me that applied focus I was looking for.
How did your career path lead you to the University of Illinois?
The academic job market is a bit like a blind date — both sides are trying to present their best selves. When I interviewed here at Illinois, there was just really good chemistry. People were genuinely curious about my research and asked thoughtful questions during my talk. That engagement stood out compared to other places.
Could you describe your primary research interests?
My work falls into two main directions, both at the intersection of Bayesian statistics and deep learning. First is Bayesian computation — traditional sampling methods have scalability issues with high-dimensional data, so I use machine learning tools to accelerate the process. This lets us handle problems that weren't feasible before, what we call simulation-based inference for models where you can't write down the likelihood. The second direction is bringing probabilistic modeling to neural networks — using Bayesian priors to enforce structure and do proper inference in these black box models.
What challenges have you faced in this research?
One big challenge is that while an approach might make sense theoretically, getting it to work in practice requires painful tuning of architectures and parameters. You need strong intuition to debug why something isn't working. Another challenge is keeping up with how rapidly the field evolves — when I started my PhD, normalizing flows were cutting edge, now it's diffusion models. There's pressure to publish before methods become outdated.
How would you describe your teaching philosophy?
I want students to find statistical tools relatable. Like when explaining treatment effects, I'll frame it in terms of marketing like, "If we offer different promotions, how do we maximize profits?" This makes the concepts click better than just equations. For my undergrads and master's students who are going into industry, it's crucial they see how these models apply to real business decisions. I always include projects where students work with actual datasets, like Amazon reviews, to extract meaningful insights.
What do you hope students take away from your courses?
I really want students to understand that statistics isn't just about prediction. Many come in obsessed with finding the best predictive model, but I push them to think about actionable questions. If you're analyzing genes and disease, are you just predicting outcomes or actually identifying causal relationships? That distinction matters in the real world.
How do you support students who are struggling?
For PhD students, I keep regular meetings to troubleshoot research problems together. I share how I debug issues — it's a skill you develop with experience. With undergrads, I try to make office hours accessible and normalize asking for help. Mental health always comes first though — if something is causing unsustainable stress, it's okay to step back.
What do you enjoy most about working with students?
Their fresh perspectives constantly surprise me. Sometimes a student will notice something in the data or code that I completely missed. Those moments when they come up with insights I hadn't considered are really rewarding. It keeps the research process exciting.
Could you share a fun fact about yourself?
I have a secret love for reality TV, especially the Kardashians. Khloe's story really resonates with me — she's been through so much but keeps going. After a long day of coding, their drama is weirdly relaxing because it's so far removed from academic life.
What are your professional goals looking ahead?
I want to move beyond purely methodological work and see our tools implemented in real applications, maybe in healthcare diagnostics. I'm also interested in developing new courses that blend Bayesian methods with deep learning, making these approaches more accessible to students.
Faculty profiles are an ongoing feature, so stay tuned to learn more about department faculty as more profiles are posted.
Ji Eun Kim is a staff writer for the Department of Statistics. If you have news to share, please contact the Statistics News Group at stat-marcom@illinois.edu