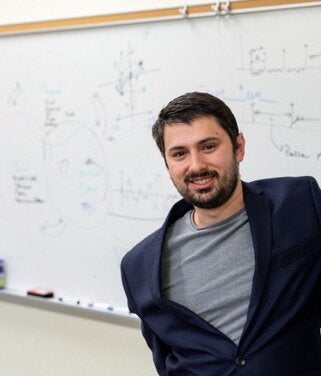
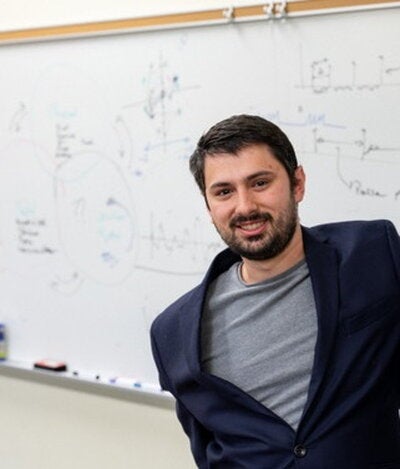
Matthew Singh, an assistant professor in the Department at the University of Illinois Urbana-Champaign, explores how nonlinear dynamics and machine learning can untangle the brain's complexity. His work develops personalized brain-stimulation strategies and tackles theoretical challenges in large-scale neural modeling, aiming to bridge math, AI, and cognitive health."
How did your career path lead you to the University of Illinois?
UIUC has a great reputation as a public research university in all STEM fields. I did my undergrad at a large public school and missed the liveliness, so UIUC was already at the top of my list. I ultimately chose UIUC statistics based upon a question at my research interview talk, which concerned biases in the long-term behavior of (nonlinear) timeseries models due to fitting-error. I had thought about these nuances intensively and couldn’t properly discuss them in a pure science/engineering/medicine department. I knew immediately this was where I wanted to be.
Can you share a defining moment or experience in your academic journey?
During grad school orientation, a professor told us “no one is an ideas-person. If you can’t show it, you have nothing.” I had previously considered myself a theoretician, so this drastically changed how I approached science.
What are your primary research interests and current projects?
My research lives at the intersection of dynamical systems, machine-learning, and neuroscience. This includes general theory of nonlinear dynamical-systems and developing machine-learning methods for understanding and predicting brain activity. My current applications are estimating large, person-specific brain models from timeseries data, and identifying optimal brain-stimulation policies to improve cognition. Coupled with this, are two theoretical questions: how to link models across spatial scales (from molecules to brain-areas) and how to frame cognitive ability as a formal, mathematical objective.
How do you see your research impacting your field or society in the long run?
Our research has a nice ability to both live quite close to the data (taking into account millimeter-anatomy, tissue biophysics) while also turning healthcare outcomes (cognitive function) into mathematical frameworks. This gives us a unique end-to-end perspective in which we can credibly turn an individual into math, (less-credibly) frame our objectives in a compatible mathematical space, and solve for optimal treatments. We are planning near-term collaborations for clinical trials. In addition, much of our research is paired with basic-science questions that can advance theoretical neuroscience. There are also potential implications for engineering. Our methodological research concerns fundamental problems in model-based engineering, but at scales in which current approaches become inapplicable. Such scales (thousands of unknown parameters, hundreds of dimensions) are currently uncommon, so time will tell how relevant our algorithms become to other domains.
What exciting research projects or collaborations are you currently working on?
We recently discovered a mathematical mechanism (bifurcations) that generates two distinct groups of people in terms of brain activity patterns. We are currently assessing the implications for person-specific treatments and cognitive ability. We are starting new research in designing person-specific brain-stimulation policies using optimal control theory.
How would you describe your teaching philosophy?
I teach programming so very hands-on—usually at least two sets of practice within each class and in my current 385 section, at least one in-class group project per week. My students come in with extremely wide backgrounds, so it’s important that everyone learns something. I like brain-teasers that can be approached at different levels—brute force for someone starting out and highly-overengineered for someone with more experience.
What do you hope students take away from your classes?
Programmatic thinking—turning arbitrary (and hopefully entertaining) challenges into fundamental operations (loops, logic etc.) Think of it like algorithms based on instinct instead of algebra.
How do you incorporate real-world applications or hands-on learning in your teaching?
I’m very hands-on, but prefer ludicrous scenarios (requiring useful algorithms) to “real-world” applications; I feel that they are much more memorable. Our current challenge-problem involves helping an evil statistician optimally cherry-pick data to support “unusual” policy positions.
What do you expect from students in your courses, both academically and personally?
Critical thinking and perseverance. I like problems that sound difficult but are approachable with the right hints. I feel these are the most rewarding but requiring trying different approaches.
How do you support students who face challenges in their learning journey?
I allow students to get help anonymously. I usually open anonymous discussion forums throughout the year to get feedback. For midterm problems I also open a separate forum for anonymous question-posting.
What hobbies or interests do you have outside of academia?
I enjoy the wilderness, particularly primitive canoeing/camping. In addition to fresh air, I like the optimization aspect—everything needs to be multipurpose and you should have several layers of contingencies.
Faculty profiles are an ongoing feature, so stay tuned to learn more about department faculty as more profiles are posted.
Ji Eun Kim is a staff writer for the Department of Statistics. If you have news to share, please contact the Statistics News Group at stat-marcom@illinois.edu