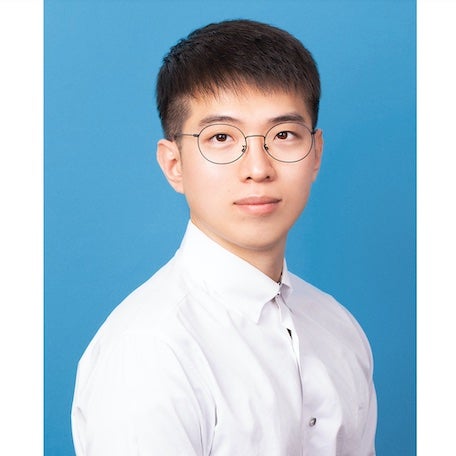
Chan Park, an assistant professor in the Department of Statistics at the University of Illinois Urbana-Champaign, studies how to better understand cause-and-effect in complex data. His research addresses challenges like missing information and interconnected data, using advanced statistical methods to improve decision-making and policy design.
What initially drew you to your field of study?
I was always interested in scientific topics. For example, I always dreamed of being a scientist, so I read a lot of books on math and science and chose my major in math and statistics. During my first year of college, I found that statistics is more fun because it pertains to real life more. There’s a lot of unknowns in this world, and sometimes you need to know a conclusion, which statistics has the answers to.
What led your academic journey to the University of Illinois?
UIUC has a strong statistics department with very active and friendly faculty, so I thought that if I joined here, I could develop further, which I couldn’t find at other schools.
What defining moments have you had in your academic journey?
I had two defining moments:
One was with my professor during undergrad in Korea, who encouraged me to pursue higher education. Before that, I wasn’t sure if I was the right person to pursue a higher degree. Pursuing a higher degree is a risk since you spend five years or potentially more doing research or writing. But once that professor recommended I do so, I became more encouraged and confident to pursue a higher degree.
The second moment was during my PhD program. A PhD program isn’t easy, and it’s like life – you’re stuck in research, imagining your future will be bright and that you’ll receive a lot of positive feedback from your peers, but the reality is that it can be very brutal. Your research can be criticized for something you thought was minor, or your work might not be well appreciated. But as time goes by, I feel my research is getting appreciated through an accumulation of publications and awards, which become great motivation for me.
What are your primary research interests and current projects?
My research is in causal inference, which is about drawing causal conclusions based on data. For example, if you wanted to study the COVID vaccine and COVID infection, that’s a good example of a causal inference problem. But in real life, data can be complex. For instance, my COVID vaccine status could be affected by those who didn’t receive a vaccine and gave me the illness, even if I followed CDC guidelines. These dependency structures can make the problem more difficult, which is one of the areas I study – drawing causal conclusions when there’s an interaction among several units.
How do you apply your research to the field of statistics?
Causal inference is multidisciplinary, and you can apply it to a broad range of applications. Among that spectrum, my work is on the theoretical end because I try to develop new statistical methodologies for learning causal effects, using machine learning, for instance. I hope to provide solid and statistically sound methodologies for statisticians and researchers.
Do you have any research projects or collaborations you are currently working on?
I work a lot with one of the educational psychologists, and it’s nice that I can provide my statistical input, and she can provide her restricted access, which I couldn’t get myself. Some of the research is about providing a mathematical curriculum where there is no bias across race, gender, or other factors you need to control. I want to provide an optimal math sequence for high school students where there’s no bias based on characteristics. This is like a causal inference problem where you want to maximize the causal effects of the math curriculum, considering the subjects of racial or gender inequality. It is statistically important but also has a high impact in terms of equal educational opportunities.
How would you describe your teaching philosophy?
I like to give the statistical ideas behind the material. I don’t want my students to simply memorize formulas, but to digest the information. I want to tell them the background story of why we do what we do, which I think makes it more interesting and provides a higher-level understanding of the work.
What do you expect from your students, both academically and personally?
I think students should be hardworking because people, or your supervisor, will know whether you're a hardworking person or not. So, even though your research or your work or report might not be the best, you’ll still get a second chance if they can tell you're a hard worker. If you’re not hardworking, you may not get a second chance. Being hardworking, whether you recognize it or not, is like insurance – it will give you an additional opportunity to survive in society.
What signs do you observe in your classroom that help you distinguish between students who are putting in effort and those who are not?
I can read the facial expressions of my students, so I can distinguish those who are paying attention from those who are not. The podium is the perfect spot to see everyone’s facial expressions. Frequent interaction is another indicator of learning whether a person is hardworking. For example, if a person comes to my office hours every week, then I know that they are hardworking.
What are your hobbies and interests?
I go to the YMCA, west of Champaign, every day and love going to parks. I also ride an electric bike.