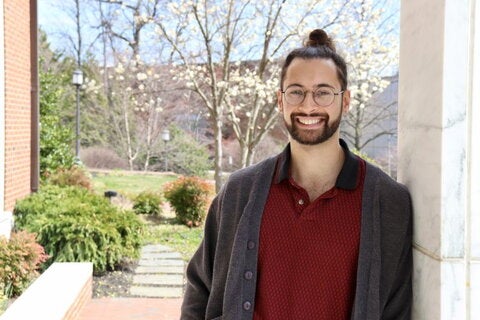
Joshua Agterberg, assistant professor in the Department of Statistics, is advancing the theoretical foundations of high-dimensional statistics and creating tools to better understand complex data structures in science and society.
What initially drew you to your field of study?
I majored in Actuarial Science and Mathematics during my undergraduate studies at the University of Wisconsin-Madison, and I always found the theoretical problems the most interesting, leading me to want to study statistics at the graduate level. I initially thought I might want to be a data scientist, but I learned during my master’s degree that there were so many unsolved problems in high-dimensional statistics, which led me to want to do research in the area.
How did your career path lead you to the University of Illinois?
After my undergraduate degree I did my master’s and PhD at Johns Hopkins University in Baltimore, Maryland, and I did a postdoc for one year at the University of Pennsylvania after that. While I loved the east coast, in my heart I am a Midwesterner, as I grew up in Milwaukee. I also liked the idea of being at a public school after spending so long at private schools, particularly a Big 10 school. After interviewing here, it was a no-brainer for me.
What are your primary research interests and current projects?
I am primarily interested in high-dimensional statistics, particularly matrix and tensor data analysis, nonconvex optimization, spectral methods, and statistical network analysis. Currently I am working on developing statistical theory and methodology for nonconvex matrix sensing and multilayer network data. I am interested in any problems that lie at the interface of optimization, matrix analysis, and statistics.
How do you see your research impacting your field or society in the long run?
I see myself as contributing to two areas of statistics: first, in my work in statistical network analysis, I believe that my work will yield a principled “pipeline” for analyzing network data of many forms, such as dynamic networks, multiple networks, and hypothesis testing for networks, all of which are relevant to fields such as sociology, neuroscience, and engineering. Hopefully my work can be used to advance research in these fields, even if not directly.
I also work on more general high-dimensional statistical problems such as spectral methods or nonconvex optimization. In my research in this area, my focus is on problems addressing the theoretical foundations of data science. It is my hope that my research will help shed light on what it is that various machine learning pipelines are actually doing, especially since they often proceed in a somewhat “black box” manner. I think this area of research is very important, as many of these algorithms are used in safety-critical systems, so understanding how they work and what can go wrong is of utmost importance.
What exciting research projects or collaborations are you currently working on?
I am most excited about my work on matrix sensing, which extends much of my previous work on spectral methods to a specific model for low-rank linear regression. While the analysis may seem only relevant to a toy model, I believe the ideas to be extremely powerful for other problems, particularly related nonconvex matrix algorithms. I am also working on a problem about multilayer networks that I believe exhibits a “statistical-computational gap,” meaning that while there is a “best possible” statistical error rate that could be achieved with unbounded computation power, it is only achievable in polynomial time when the signal strength is above a certain threshold.
How would you describe your teaching philosophy?
I believe in developing strong fundamentals and conveying intuition. Statistics is not a field that should be formula-based, and having strong principles develops statistical intuition. When faced with a difficult problem, students should be able to attack it from first principles.
What do you hope students take away from your classes?
I hope that students can walk away from any class having developed good statistical intuition for attacking complicated problems. Or that they should rarely be using a T-test in the real world.
What do you enjoy most about working with students?
For PhD students, I enjoy seeing their journey from beginning student to expert in their field. My favorite thing with any new PhD student is being told I’m wrong about a research problem – this usually means that the student knows more than me.
For undergraduates, I enjoy seeing them develop intuition about statistical concepts. I remember being confused about various facts while I was in undergrad, and seeing it “click” for someone struggling with a concept is one of the most enjoyable parts of the job.
What’s a fun fact about you that most people don’t know?
My wife and I have the same birthday! Same year and everything!
What do you hope to achieve in the next few years, both in research and teaching?
In research I am hoping to provide a novel set of tools and techniques for analyzing network data, and I hope to marry this with my more fundamental research in spectral methods, nonconvex optimization, and high-dimensional statistics. I often like to analyze “toy” models and provide rigorous theoretical guarantees for these models, but I also like to use what I learn from these toy models to help advance statistical methodology and algorithms.
In teaching I am hoping to help develop a course on high-dimensional statistics for PhD students, which I believe to be essential for modern theoretical research. I am also hoping to teach a topics class on my research area.
Faculty profiles are an ongoing feature, so stay tuned to learn more about department faculty as more profiles are posted.
Ji Eun Kim is a staff writer for the Department of Statistics. If you have news to share, please contact the Statistics News Group at stat-marcom@illinois.edu