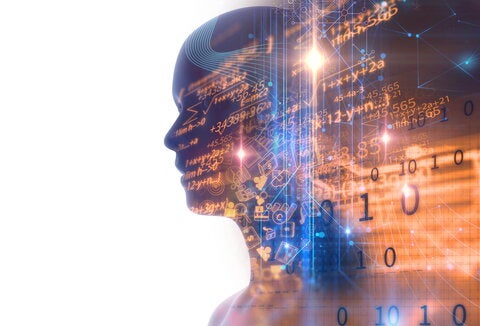
“Our lives and the things that we value as humans are all impacted every day by the decisions and predictions made by algorithms which are typically at least explicitly only optimizing for just the single human value: accuracy,” stated Tori Ellison.
Tori Ellison has been a teaching assistant professor in the Statistics department since 2020. She currently teaches STAT 207: Data Science Exploration, STAT 430: Data Science Ethics, and STAT 437: Unsupervised Learning. With a strong interest in mathematical optimization, she obtained her PhD in Operations Research at North Carolina State University. Additionally, Ellison has also served as an advisor in the department for 2 years for students undergoing their master’s program.
“I’ve always been interested in widening the framework with which Statistics students view and understand traditional statistical concepts like predictive modeling and unsupervised learning,” expressed Ellison.
Predictive modeling is a process that uses models, such as logistic regression models, to predict probable outcomes with the given set of input data. This process utilizes a mathematical optimization framework, in which Professor Ellison explained it as, a set of variables that we select to either maximize or minimize function(s) to get our desired results. They can be used as a set of constraint functions to limit the possibilities that these variables can take on as well. Ellison noted that many students may not realize that you don’t need to limit yourself to optimize for just one function. “You can actually choose to optimize a combination of functions in which would represent a combination of human values as well,” stated Ellison. On the other hand, unsupervised learning is a branch of machine learning that uses algorithms to find patterns and cluster unlabeled datasets. These algorithms can also reveal the hidden insights in high-dimensional unlabeled datasets.
Furthermore, Ellison shares some theories regarding how developing reliable text mining AI assistance tools can benefit an advisor’s role, based on her personal experience when she was an advisor. She theorizes that utilizing AI assistance tools can reduce the redundancies of the common inquiries made by students that get registered into the system. These tools in theory can optimize the system by grouping common topics that students inquire and allow for advisors to better prepare for their sessions and have time for more in-depth and personalized advising sessions. Another theory Ellison shared is that text mining AI tools could potentially be able to analyze lecture notes from all departmental classes, enabling the identification of both overlaps and deficiencies of content. This would benefit students because these tools may be able to recommend an ideal course selection for students with specific career aspirations.
In addition to these potential applications of AI tools, Ellison stated that she aims “to build a data science ethics course that focuses on small-scale algorithmic decision-making that can be made any day by an individual data scientist.” This approach differs from the traditional case-study based approach that focuses on examining problematic instances of an algorithm in practice and the potential actions of what CEO or policy makers could do or could have done instead. Ellison wanted to transition from the traditional approach due to recent claims made by researchers who “called into question the efficacy of these traditional courses, especially when it comes to cultivating the skill of moral sensitivity amongst students taking the class,” stated Ellison. Ellison further explained that “This is the skill in which a person can first detect whether there is an ethical dilemma at play in a given decision. Researchers have argued that students find it harder to relate and learn moral sensitivity from the traditional case study approach that focuses on high-level ethical data decisions made by CEOs or policy makers.”
Notably, Professor Ellison’s STAT 430 course exposes students to state-of-the-art algorithms for quantifying specific values and integrating them into mathematical optimization frameworks. Her course utilizes Python and R-based packages created by IBM researchers that provide user-friendly functions for implementing these cutting-edge algorithms. This semester, Ellison plans to incorporate some ChatGPT usage into her coursework to demonstrate how beneficial AI tools like ChatGPT can be used and how it can also be unreliable. Most importantly, Ellison cares that students who do choose to use ChatGPT, are using it responsibly. To ensure that students are using AI tools effectively and responsibly, she will be implementing a one-on-one checking mechanism in which she evaluates the extent to which students are able to explain their problem-solving thought processes as well as demonstrate a solid understanding of the material.
Ellison stated that, “Articulating your thought process when it comes to data modeling and coding is most likely what you will be asked to do in your data science interviews. Practicing this in college is a useful career development skill to hone.”
By the end of the course, Ellison hopes that her students will leave with the proficiency to implement these algorithms in their data science workflows and be able to evaluate its ethical implications. She hopes that she has helped them hone their skills of being an “algorithmic translator” which is to bridge the gap between algorithmic decision-making processes affecting society and the members impacted as an outcome.
Gianna Pham
2023-10-06
Gianna Pham is a staff writer for the Department of Statistics. If you have news to share, please contact the Statistics news group at stat-office@illinois.edu.